Robin Msiska
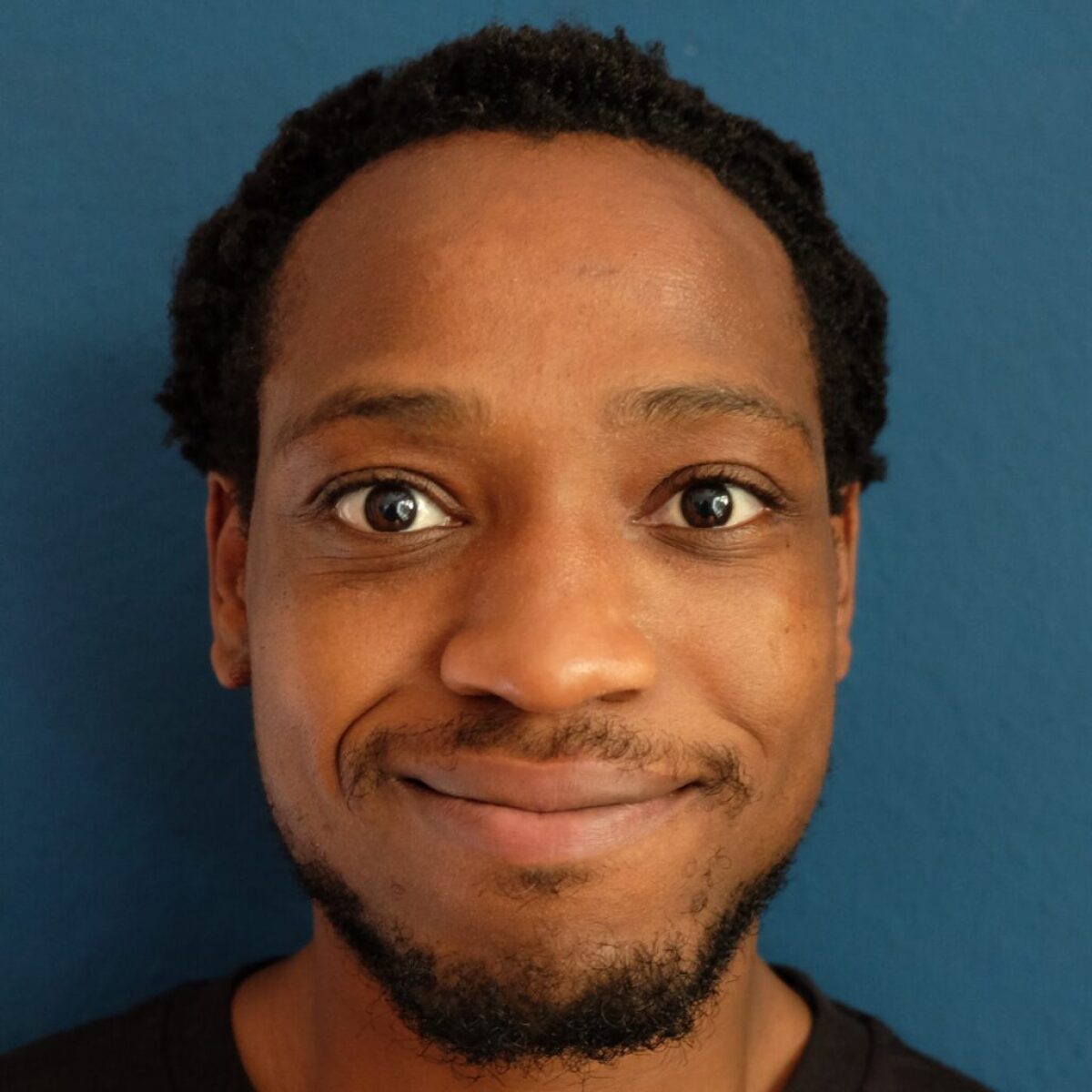
Robin Msiska
Positions | PhD Candidate |
robin.msiska@uni-due.de | |
Phone | 0203-379-4719 |
Office | MG 385 |
Address |
University of Duisburg-Essen |
Contact | Researcher-ID: FQF-3718-2022 |
Fakultät für Physik, Theoretische Physik
Address
Lotharstraße 1
47057 Duisburg
47057 Duisburg
Room
MG 385
Phone
E-Mail
Functions
-
Wissenschaftliche/r Mitarbeiter/in, Arbeitsgruppe Prof. Everschor-Sitte
Current lectures
No current lectures.
Past lectures (max. 10)
-
2021 WS
The following publications are listed in the online university bibliography of the University of Duisburg-Essen. Further information may also be found on the person's personal web pages.
-
Audio Classification with Skyrmion ReservoirsIn: Advanced Intelligent Systems Vol. 5 (2023) Nr. 6, 2200388Online Full Text: dx.doi.org/ (Open Access)
-
Perspective on unconventional computing using magnetic skyrmionsIn: Applied Physics Letters (APL) Vol. 122 (2023) Nr. 26, 260501Online Full Text: dx.doi.org/ (Open Access)
-
Spatial analysis of physical reservoir computersIn: Physical Review Applied Vol. 20 (2023) Nr. 4, 044057Online Full Text: dx.doi.org/ (Open Access)
-
Nonzero Skyrmion Hall Effect in Topologically Trivial StructuresIn: Physical Review Applied Vol. 17 (2022) Nr. 6, 064015Online Full Text: dx.doi.org/ (Open Access)